350 rub
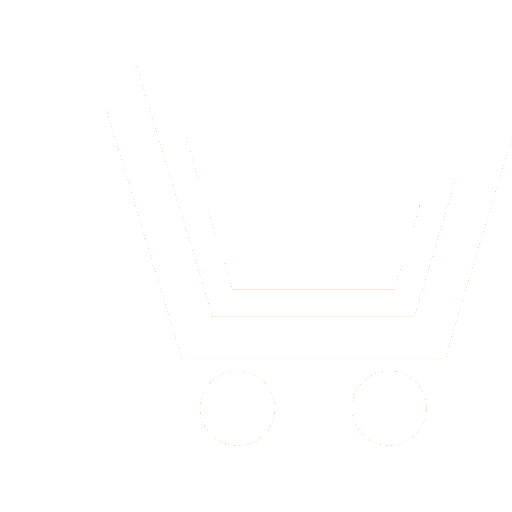
Journal Dynamics of Complex Systems - XXI century №3 for 2013 г.
Article in number:
Business process modelling method based on event logs
Authors:
I.A. Khodyrev
A.V. Boukhanovsky
A.V. Boukhanovsky
Abstract:
Modern information systems provide data about processes inside organizations or communities in the form of so-called event logs. Business management information systems, such as ERP, WFM, CH and CRM systems allow creation of business process models, control, regulate process flow, and automatically gather information related to the process in event logs. Also as resources about processes collaborative systems could be used (for example problem oriented environments such as eScience, eEngineering or social networks from the web). Gathered information allows manual analysis of processes as well as automated building of process models for considered object. There could be a variety of application for such models, and in presented paper we only focus on indicator prediction task. In presented paper we propose an approach for automated building of simulation models of processes based on event logs, which uses information about context of process execution, for the task of indicator prediction. Different process related variables could be considered indicators: time consumption on some task class, number of process initializations per period, average load on some type of resource etc. Simulation model building consists of 4 main stages: event log filtering and Petri Net model extraction, context model building, data dependencies inclusion into process model, model integration into simulation model. After simulation model is built simulation exepriments could be conducted to obtain values for target indicators.
Proposed approach has several important differences from the state of the art methods:
1. Usage of combined process mining algorithm, allowing to obtain models from real-life event logs.
2. A number of significant feature classes was used on the stage of data dependencies inclusion.
3. Nonparametric methods used for data dependencies formalization.
4. Accounting data aging.
Paper contains results of experiments with synthetic models using different data dependencies formalization algorithms.
Pages: 46-50
References
- Semenov A. V., Buxanovskij A. V. Metrologicheskij analiz v soczial'ny'x setyax // Izvestiya vy'sshix uchebny'x zavedenij. Priborostroenie. 2011. T. 54. № 3. S. 85 - 86.
- W.M.P. van der Aalst, A.J.M.M. Weijters, and L. Maruster. Workflow Mining: Discovering Process Models from Event Logs. IEEE Transactions on Knowledge and Data Engineering, 16(9), 2004. R. 1128 - 1142.
- Xody'rev I. A., Popova S. V. Sravnenie algoritmov Process Mining dlya zadachi poiska modelej proczessov // Nauchno-texnicheskie vedomosti SPbGPU. 2011. № 6.
- Xody'rev I. A., Popova S. V. Obnaruzhenie razvilok v modelyax proczessov. Nauchno-texnicheskie vedomosti SPbGPU. 2012. № 3.
- Constiantis S. B. Supervised machine learning: a review of classification techniques. Informatica. 2007. T. 31.